Automating classification with artificial intelligence (AI)
Artificial intelligence (AI) applies to a wide range of fields, yet it remains an abstract concept for many people. The use cases seem magical, but often lack the concreteness to enable organizations to project themselves easily onto the problems they face. But there’s no denying that this subject is becoming increasingly important – even more so since the advent of generative AI like ChatGPT. Our customers are increasingly curious to discover how AI can help them in their work.
A recent example is automatic data classification. This practice involves developing algorithms capable of sorting and organizing large volumes of data according to certain criteria. In the context of automatic classification, AI uses machine learning (ML) and deep learning (DL) techniques to analyze and categorize data automatically. These techniques enable AI to learn from large training datasets, in order to develop models and algorithms capable of generalizing the classification of new data. The innovative approach fully exploits the capabilities of these new technologies, effectively replacing the more traditional way of development based on a faithful and precise definition of the rules linking a piece of data with its reference category.
This has many advantages, such as speeding up the classification process by rapidly processing large quantities of data, or identifying complex relationships between data, which may be difficult for the human eye to detect.
Use cases
Automatic data classification is applicable to a wide range of fields, including healthcare, finance, e-commerce and scientific research. For example, in healthcare, AI can be used to automatically classify medical images, test results or medical records, enabling improved diagnosis and medical decision-making.
Product categorization
With numerous customers in the distribution and retail industry, Synchrotech has evaluated and validated a use case that meets the needs of the sector. Our solution Product Categorizer (see our ML Library offering) brings added value to the categorization of products specific to an organization.
Based on an ML model, our standardized solution automates the process (category deduction) and makes it more reliable (error reduction). The category tree is defined upstream, and is used to train the model on the basis of existing product data. Applied to our customers’ contexts, the solution offers the following benefits:
- Reduce data handling costs;
- Increased sales thanks to better visibility of products in the right category (e.g. for e-commerce);
- Optimization of assortment and improvement of management processes (purchasing, inventory, logistics, etc.).
The automatic classification process consists of 6 steps, as shown in the diagram below. The diagram also shows an example of the results that will be given to users (in step 5) for verification and approval.
What are the steps in our process?
Six stages enable the team to train, test and validate the model, including a verification and approval stage to guarantee the quality of the data propagated to the applications.
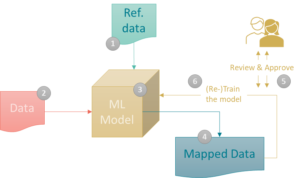
How does the team monitor the results?
Our ML model automates the Matching process and, as a result, produces a reliable dataset submitted to the team for approval of the right match among the three most likely recommendations.
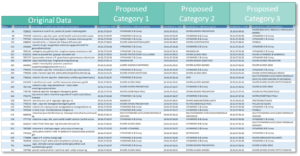
Other examples of use
Properly mastered, the category approach enables manufacturers to differentiate themselves from their competitors and gain an advantageous position in the market. In addition to the case presented above in the field of Category Management, the optimization of which makes it possible to develop the sales of a product category and that of a brand, other examples can be cited.
“Product” data is highly structured, often standardized through the use of a Product Information Management (PIM) solution, or even standardized for the exchange of product catalog data (e.g. BMEcat). In e-commerce sales, the use of product taxonomy helps search engines (e.g. Google) to determine the terms for which products will be displayed, enabling your ads to reach the right target audience. Accurate product classification enables companies to better understand customer preferences and behaviors. By using category-based recommandation techniques, companies can suggest similar or complementary products to their customers, which can boost sales and improve customer loyalty.
Another example is the Advance Recycling Fee (ARF). This is levied on all electrical appliances when they are sold, rented or leased. Depending on their designation, assignment to the correct category ensures the correct application of the ARF in the product tariff. Indeed, AI can be used to automatically classify products into appropriate categories according to their characteristics, composition or material. This enables the amount of Advance Recycling Fee applicable to each product to be determined quickly and with a marginal error rate.
Conclusion
The use of AI techniques can help automated the classification process and improve the accuracy of the results. It should be noted, however, that classification remains a challenge in some cases, particularly if the data may belong to several classes (or categories), or if new classes need to be created. But even in the latter scenario, AI has the ability to create new categories when none match the data provided. This can be achieved using machine learning techniques, in particular unsupervised learning. Human validation remains necessary, however, to guarantee the relevance and quality of the new categories generated. We recommend this to our customers, in order to correct biases and take into account the context to ensure ethical results, thus reinforcing the confidence of end-users.
Curious about the benefits AI can bring to your business? Then get in touch with us! Our Data Science experts will be delighted to discuss this with you!